
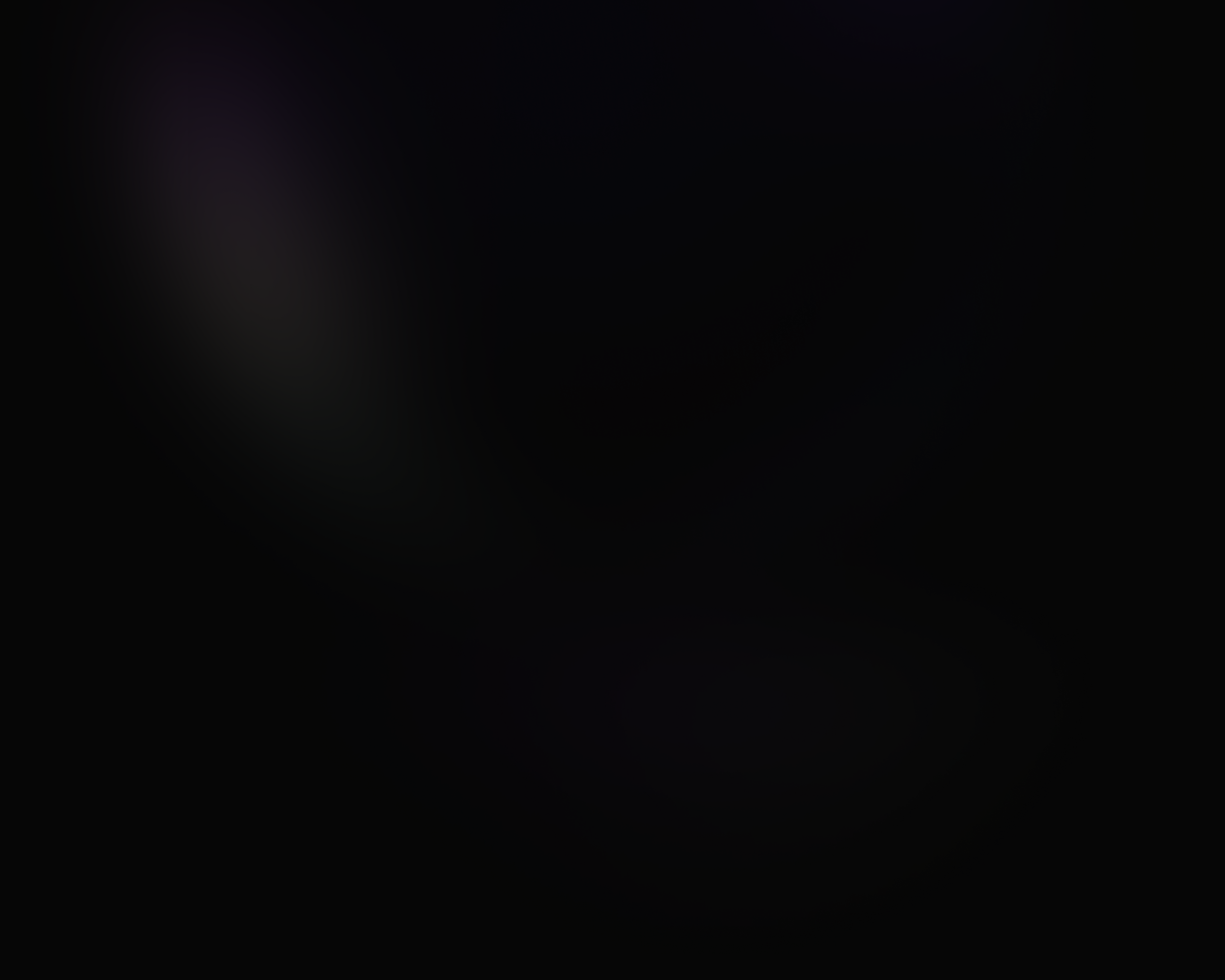
Overview
Synthetic Data is Key for Improving Kaleido AI's Models
Headquartered in Vienna, Austria, Kaleido AI’s mission is to make complicated tech simple. By creating tools that simplify and accelerate workflows, foster creativity, and enable others to bring new ideas to life, the company provides everyone - from individuals to businesses of all sizes - access to the latest advancements in visual AI.
In 2019, Kaleido AI introduced remove.bg, the automatic image background remover, built to eliminate manual work and complex tools with high learning curves. In 2020, the team launched Unscreen, an online software that removes video backgrounds in no time. These simple but effective tools dramatically increased the speed at which users could achieve their goals. As a result, their popularity skyrocketed, which ultimately led to Canva acquiring Kaleido AI in early 2021.
Later that year, Kaleido AI launched Designify, the AI-powered tool that creates automatic designs for individuals, car dealerships, e-commerce websites, and more.
Kaleido AI’s incredibly effective and accurate tools use machine learning algorithms to automatically detect objects and backgrounds and return background-free photos and videos. Through easy-to-use APIs and plugins, developers and editors accelerate their workflows using Premiere Pro, After Effects, or other editing software.
Kaleido AI uses Scale Synthetic for synthetic data generation, edge case identification, and Scale’s machine learning expertise.
The Problem
Kaleido AI Needed Better Data to Improve Segmentation for Challenging Edge Cases
Kaleido AI takes an AI-first approach to its visual editing tools, with a talented team of ML engineers on staff. Enabling simple, automated visual editing tools that work requires excellent machine learning models and excellent machine learning models need a large volume of high-quality data.
However, Kaleido AI encountered several edge cases in a specific segmentation task where their model performed poorly. Collecting and labeling tens of thousands of real-world images with a large diversity of patterns, images, backgrounds, and textures was difficult. Open datasets did not have enough high-quality images of this particular class.
In the initial attempt to solve this issue, Kaleido AI relied on real-world data to train its segmentation models. Unfortunately, collecting and labeling a large amount of diverse real-world data was complex, resource-intensive, and costly. After this initial effort, the team determined that instead of spending the time and resources to develop their models with real-world data, they should consider using synthetic data to augment their dataset. So, they sought help from the experts in synthetic data at Scale AI.

The Solution
Synthetic Helps Kaleido AI’s ML Team Prioritize and Generate Better Data to Improve Model Performance on Business-Critical Edge Cases
Kaleido AI asked Scale to help them generate synthetic data to help them continue to improve their model performance on object identification and improve the IoU of their model predictions. Kaleido AI had plateaued at an IoU of 0.657 with real-world data and needed to improve its model continuously.
Scale’s ML Engineers started by analyzing Kaleido AI's sample data and model inferences in Nucleus, Scale’s data curation platform. Scale quickly identified that the model was performing poorly in segmenting objects in images with complex patterns, the objects were shaded or transparent, or where there were shadows in the backgrounds of the scenes. Scale focused on these edge cases and generated a sample of 2,650 images of synthetic data with varied lighting, textures, and patterns. However, this first pass was not sufficient to meaningfully improve model performance.
The Scale team recognized that they needed more data specifically targeted at problem edge cases to improve model performance substantially. So the team did a deep dive into Nucleus to curate data to further identify these problem edge cases. They also introduced the ability to visualize Scale’s synthetic images compared to real images in 2D space. To do this, the Scale team first passed all the images to a RESNET 101 backbone and took the vector embedding of each image at various layers before passing them to t-SNE. Then, the team visualized which synthetic images were furthest in 2-dimensional space from real images and which real images formed a distinct and unaddressed cluster of images. Using this method, the Scale team identified the real images furthest from synthetic images and prioritized these edge cases.
This analysis revealed that Scale needed to include more textured/patterned objects and a wider variety of object types in the synthetic data distribution.
Upon further investigation, the clump of red images towards the center of the plot were patterned objects.
This analysis identified additional patterns and other edge cases on which to focus. We also determined that white objects on white backgrounds with dirt or speckled backgrounds or where there are two layers of background (i.e., rooms with doors) were causing poor model performance.
The Scale team now had a clear understanding of what was needed to improve model performance and set about generating a larger dataset with data targeted at addressing these edge cases. In total, Scale generated 14,583 synthetic images covering a total of 12 categories covering patterns, various objects, backgrounds, and textures. With this targeted synthetic data, Kaleido AI achieved an IoU of 0.794.
The Result
With Scale Synthetic, Kaleido AI Improves Model Performance While Focusing on Building Their Core Product
Today, Kaleido AI’s model is built on five hundred real-world images and almost fifteen thousand synthetic images from Scale. Kaleido AI needed to improve its model performance and efficiency to enable visual AI for everyone. Scale Synthetic helps Kaleido AI achieve its goal by enabling continuous and efficient model performance improvement on target edge cases.
Looking forward, Kaleido AI intends to continue to increase the amount of synthetic data in its dataset, using Scale’s Synthetically generated data to improve its models. There are many use cases that Kaleido AI is considering focusing on next, and Kaleido AI will continue to look to Scale to help them improve their model performance.
With Scale Synthetic, Kaleido AI has reduced the time and effort involved with synthetic data curation, so the team can now focus on improving its core application.
