
Revisiting the Superficial Alignment Hypothesis
Mohit Raghavendra°1 , Vaskar Nath2 , Sean Hendryx2
1Georgia Institute of Technology, 2Scale AI, °Work conducted while at Scale AI
The Superficial Alignment Hypothesis suggests that most of a language model's knowledge and skills come from its initial training, while post-training is about giving a model the right style and format. This study revisits this hypothesis by empirically studying the scaling behavior of post-training with increasing finetuning examples and evaluating them using objective task-specific standardized benchmarks for skills like math, coding, and reasoning.
The findings show that, similar to pre-training scaling laws, post-training task performance scales as a power law against the number of finetuning examples. For complex tasks like math and reasoning,a handful of post-training examples merely align the model stylistically but do not saturate performance on the benchmarks; more extensive examples lead to meaningful improvements in domains like multi-hop reasoning. This indicates that models aren’t strictly limited by their initial training knowledge. With the right post-training, models can learn and apply new information to better tackle complex questions.
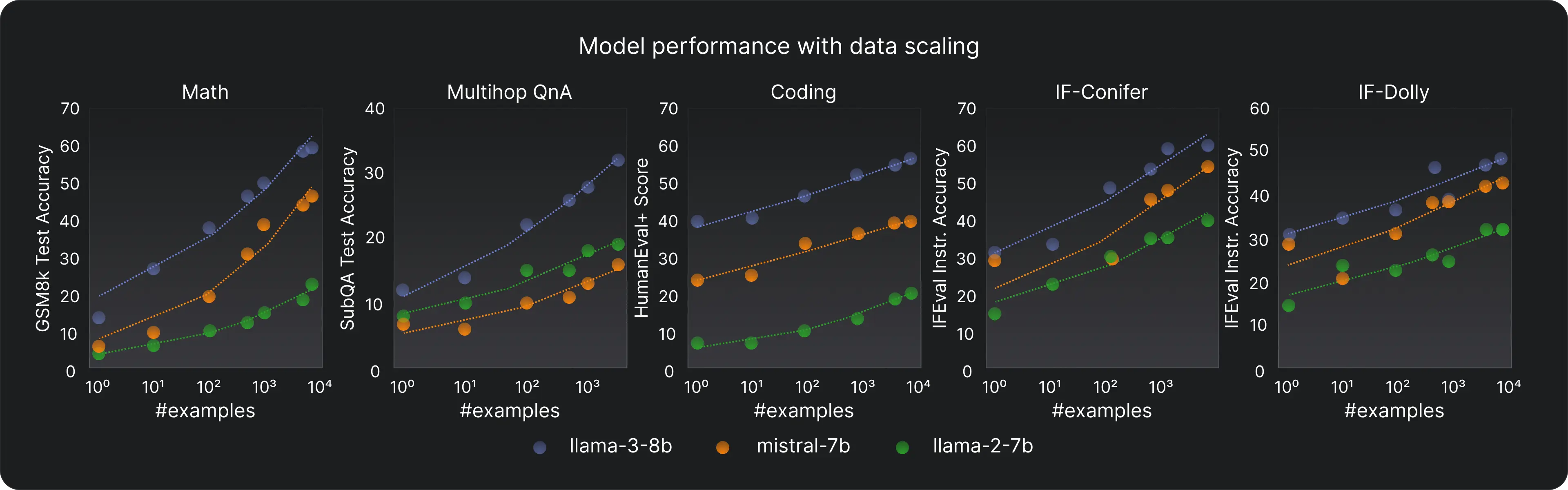
Performance improvements as finetuning data is scaled up, for models in the sub-10 Billion parameter range. The points are fitted with a power law curve of the form P ∝ D1/b . Model performance consistently scales in a power law fashion, across model families.
These findings suggest that evaluating models using a single preference vote can be misleading for reasoning tasks, especially those with an objective component. A holistic evaluation program covering both human preference alignment and rigorous reasoning benchmarks is required to valuate general purpose frontier models. This suggests the Superficial Alignment Hypothesis may oversimplify how language models learn and develop skills.
Bibtex Citation
@misc{raghavendra2024revisitingsuperficialalignmenthypothesis,
title={Revisiting the Superficial Alignment Hypothesis},
author={Mohit Raghavendra and Vaskar Nath and Sean Hendryx},
year={2024},
eprint={2410.03717},
archivePrefix={arXiv},
primaryClass={cs.CL},
url={https://arxiv.org/abs/2410.03717},
}