
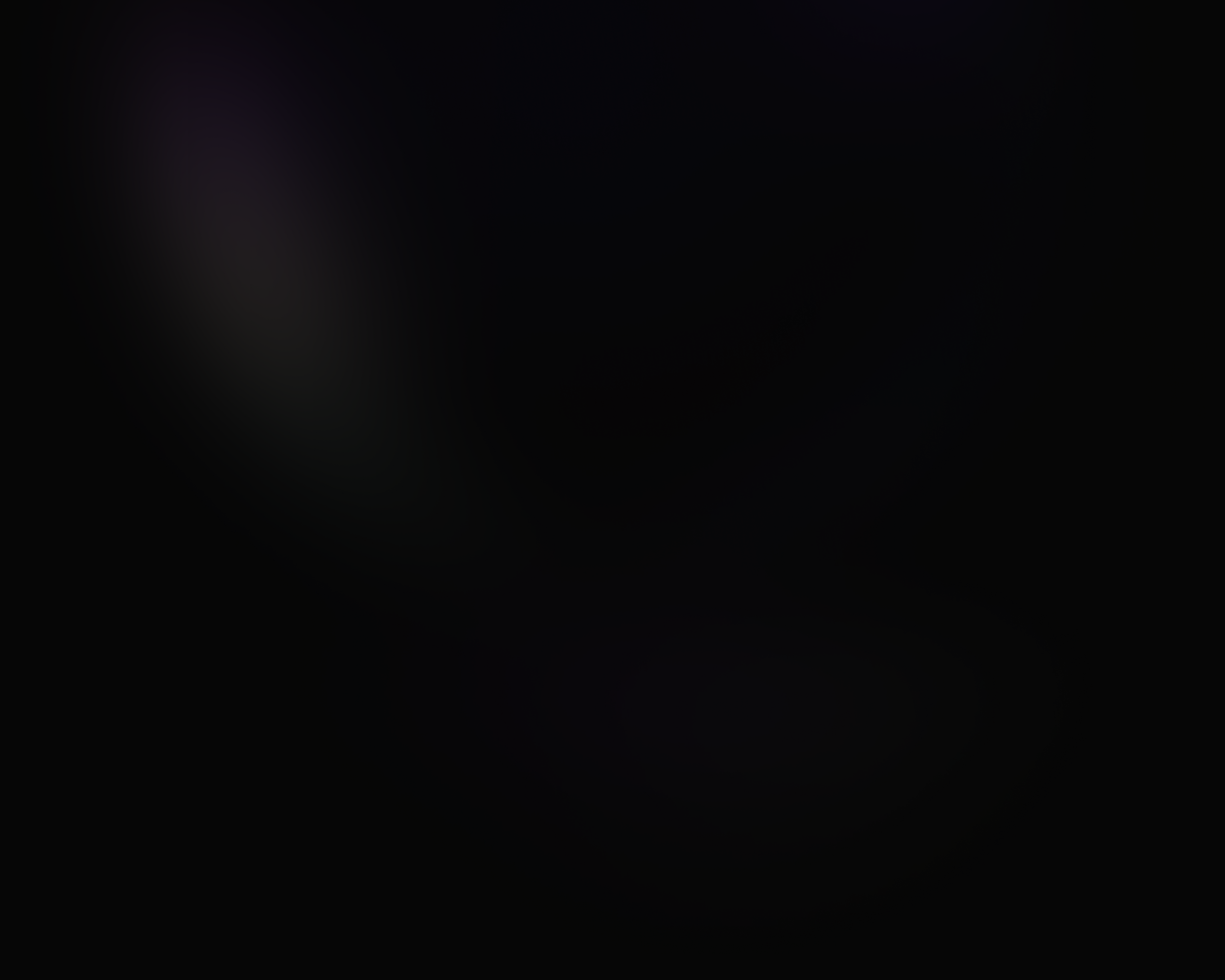
Overview
High-Quality Annotations are a Vital Part of Rapidly Improving Models
The Problem
Ambi Robotics Needed a Scalable Approach for Data Annotation
Training a well-performing ML model to perform a task as complex as pick-and-place requires a lot of annotated data. While initially Ambi Robotics was managing the annotation process in-house, the approach was simply not scalable for the amount of data they needed. When working with new clients and locations, for example, Ambi Robotics would sometimes see lower pick-and-place success rates, simply because the environment looked different. The best way to improve performance was to mine data from the new location, annotate it, and then retrain their ML model.
The company didn’t have the infrastructure to process this large quantity of data on a recurring basis, however – Regularly generating thousands of labels in 24-48 hours internally wasn’t a feasible solution. To provide all of their customers with the best possible experience, they needed a more scalable approach to data annotation.

The Solution
With Scale Rapid’s Tooling and Infrastructure, Ambi Robotics Obtains High-Quality Annotations Quickly
Now, when Ambi Robotics works with a new client, they can outsource the data annotation process to Scale Rapid, quickly obtaining the annotations they need to improve their ML models. Scale Rapid offers a simple step-by-step approach for setting up the annotation process, making it easy for customers to set up a new task in just minutes.
In order to provide high-quality data, Scale Rapid builds quality evaluation into their pipeline through batch annotation. Scale Rapid produces a calibration dataset to identify gaps in the labeling instructions, until the customer is satisfied with the data calibration. After calibration is complete, Scale Rapid produces a production batch for the client.
Scale Rapid has also provided Ambi Robotics with a reduction in lead time – While Ambi Robotics’ internal annotation process could sometimes take weeks, Scale Rapid consistently delivers production-ready results within 1-2 days. When assessing data annotation quality, Ambi Robotics has found that the results delivered by Scale Rapid are consistently on-level with their in-house annotation, while being produced using robust and scalable infrastructure.

The Result
Ambi Robotics Provides New Customers with Better Robotics Performance
The real world is filled with edge cases, so Ambi Robotics is always looking to increase their robotic systems’ pick success rate. Improving this metric provides customers with a better pick-and-place experience, and since Ambi Robotics began working with Scale Rapid, the model improvement process has become much easier.
Now, when the company sees degraded model performance at a new customer location, they can simply mine the relevant data and send it to Scale for annotation. Then, they can retrain their ML models using these data annotations, making the pick-and-place performance of their robotics systems more robust to new clients and locations.
After incorporating Scale Rapid into their production pipeline, Ambi Robotics can obtain high-quality data annotations quickly and easily, making it simple to use continuous learning to improve their system’s pick success rate.
