
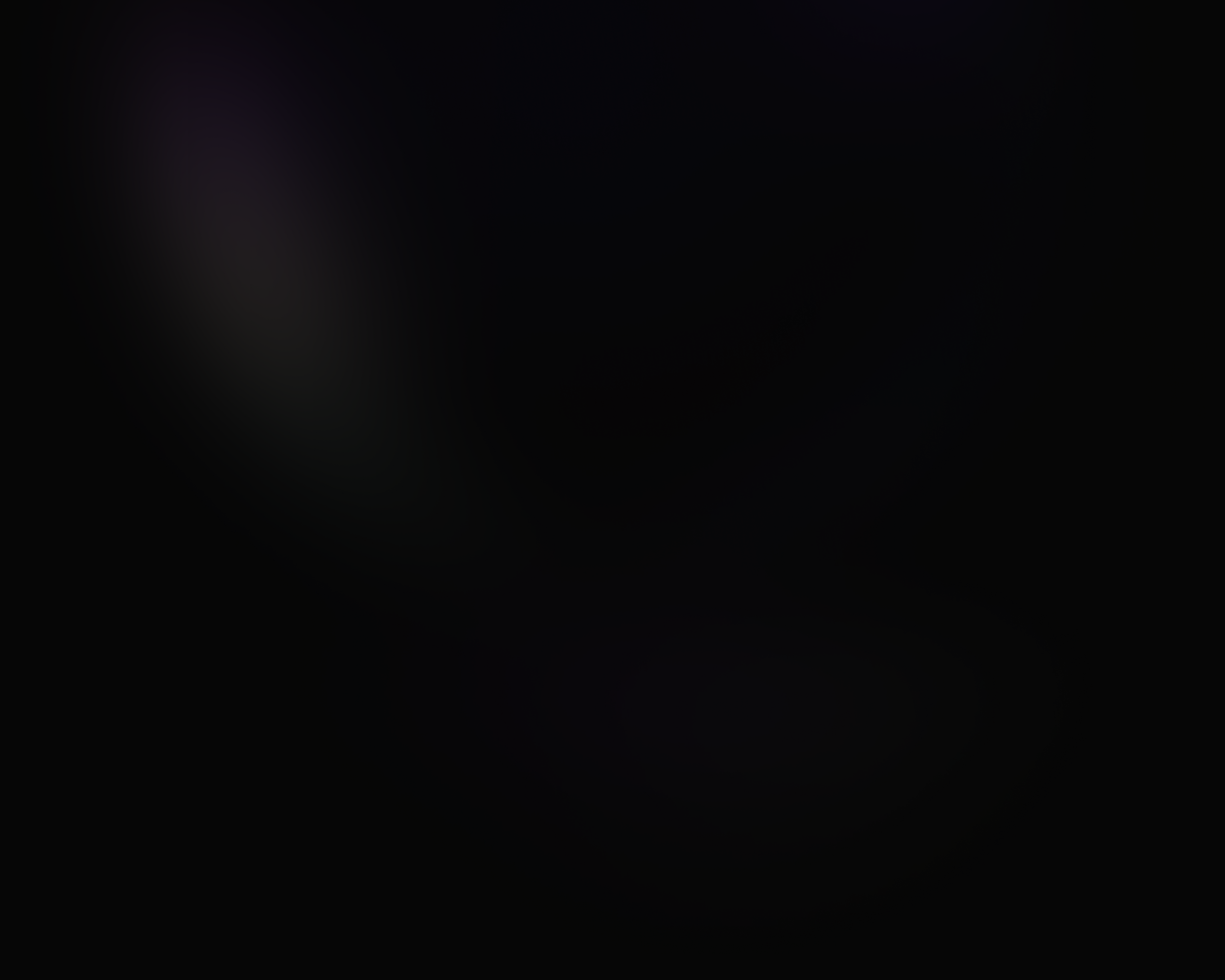
Overview
With Scale Rapid Annotating their Data, the Researchers in the Datta Lab Can Focus on the Science
The Datta Lab at Harvard Medical School is studying the neural mechanisms associated with behavior in rodents. To conduct their research, they place mice in an experimental rig, and then record each mouse’s behavior using cameras. They also measure each mouse’s neural activity using neural implants. They can then analyze this data to examine the link between neural activity and behavior, as well as to advance their understanding of specific brain areas of mice. Actually analyzing this data, however, is a time-consuming process – To interpret the behavioral data, the researchers must first label the poses of the mouse over time. While the lab can train machine learning (ML) models to perform this automatically, a large amount of video footage needs to be manually annotated first. This annotation process detracts from the time that researchers could instead be spending on the aspects of research that require their expertise. The Datta Lab at Harvard Medical School is now working with Scale Rapid to get the annotations they need. Instead of spending weeks labeling their data, they can pour their full attention into advancing their research.
The Problem
The Datta Lab Needed to Speed Up their Data Annotation Process
Analyzing a mouse’s behavioral data requires annotators to take the raw video of a mouse and extract detailed kinematic variables that describe its body’s position at each moment in time. In particular, researchers are often interested in the mouse’s joint position over time, such as the location of its toes, knees, and hips.
Before researchers can train a model to detect these key points, however, they first need to build a training set. This is not a quick process – An annotator needs to sit in front of a computer for hours labeling hundreds of images. Before the Datta Lab worked with Scale Rapid, this annotation was performed entirely in lab. This consumed a huge amount of time that could otherwise have been spent on the more salient aspects of research.
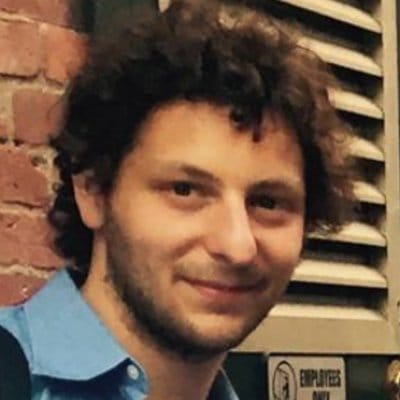
The Solution
Scale Rapid Quickly Provides the Datta Lab with the Annotations They Need to Advance their Research
Now that the Datta Lab is working with Scale Rapid, they no longer need to annotate their data in-house. Instead, they can send their raw videos of mice to Scale Rapid and quickly get quality annotations in return. These annotations provide the lab with the information they need to train their ML models for automated pose tracking.
Obtaining annotations from Scale Rapid saves the lab weeks of labeling time, allowing researchers to spend their time more effectively. Working with Scale Rapid also allows the lab to acquire a higher volume of annotations than they could ever accomplish in-house. Having a large volume of high quality annotations makes it easier than ever for the lab to train high-performing ML models, while also freeing up researcher time.
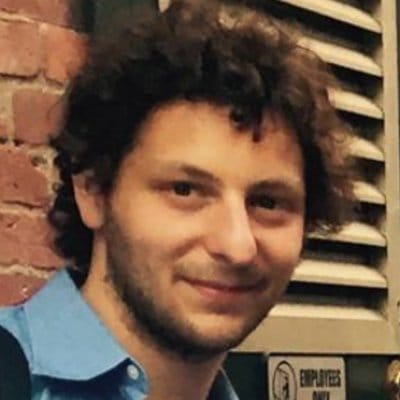
The Result
With Scale Rapid, the Datta Lab gets Better Research Outcomes Faster
Instead of spending weeks annotating data, the lab now sends their data to Scale Rapid and gets quality annotations as quickly as overnight. The easy access to data annotations has lowered the barrier to training new models, so the lab has been able to try out new ideas and iterate more rapidly. Now, if a researcher needs to collect new training data after updating an experiment, or if they need to track a different part of the mouse in existing data, they can easily send their footage to Scale Rapid for new annotations. This makes it possible for the lab to explore possibilities that simply weren’t feasible before.
Additionally, the ease with which the lab can obtain high volumes of data annotations has made it easier to produce high-performing ML models. Having a large amount of data can reduce the need for model fine-tuning – The majority of edge cases are naturally handled by the model, so researchers don’t need to spend weeks adjusting their model for these shortcomings. By using Scale Rapid, the Datta Lab can now spend more of their time advancing their research in neuroscience.
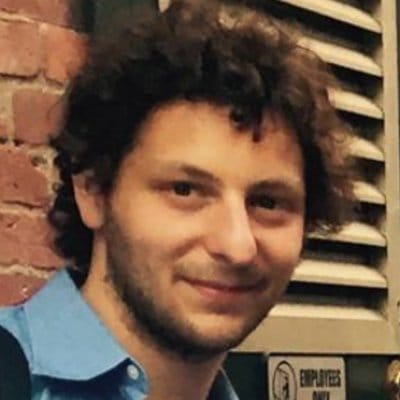