
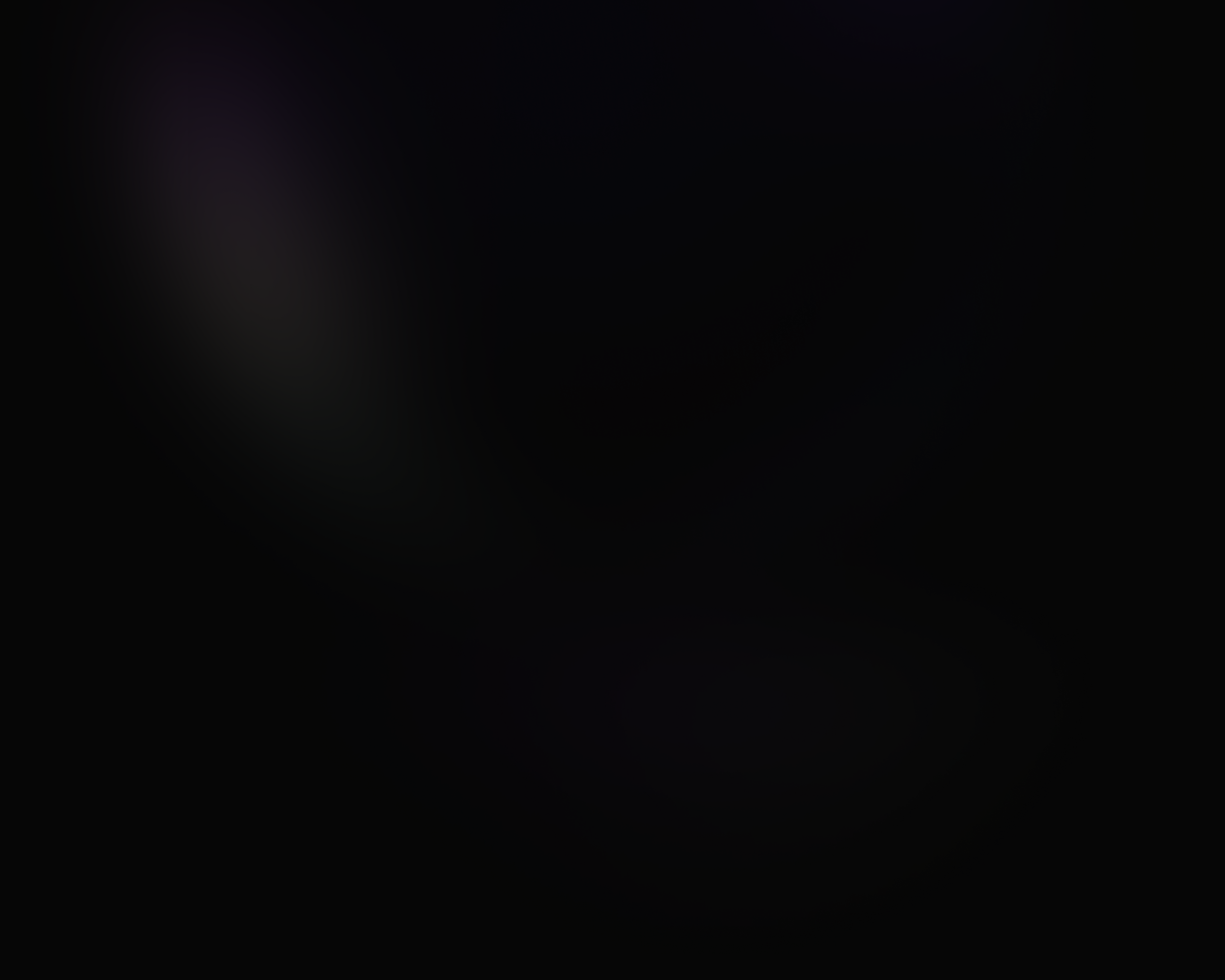
Overview
Nucleus Enables Rare Class Mining—Essential for Safe Autonomy.
Headquartered in Mountain View, CA, Nuro exists to better everyday life through robotics. The company’s custom electric autonomous vehicles are designed to bring the things people need—from produce to prescriptions—right to your home. Its R2 robot was the first completely autonomous, zero-occupant on-road vehicle approved for commercial delivery by the U.S. Department of Transportation. Last April, the company partnered with Domino’s to autonomously deliver pizza to customers in Houston and last June, Nuro announced a new partnership with FedEx to add to a growing roster of some of the largest brands in the US. Nuro has piloted autonomous, eco-friendly local delivery for communities in Texas, Arizona, and California, aiming to make streets safer and cities more livable.
Nuro’s approach to building a custom-goods only vehicle allows it to turn safety innovations that have traditionally been focused internally on passengers (seatbelts, airbags, etc) inside out to prioritize vulnerable road users such as bikers and pedestrians. The R2 robot features 360° and thermal cameras, as well as lidar and radar for a rich representation of the road. Nuro builds its own HD maps, and its AV stack combines engineered and machine-learned components for maximum robustness. A redundant collision prevention system provides an additional layer of safety. Nuro uses Scale to label both 2D and 3D data, generate and label HD maps, curate its training data, and identify new edge cases to solve with new labels and better models.
The Problem
Nuro Needed Better Tools to Identify Infrequent but Meaningful Scenarios in Training Data.
Nuro faced the daunting task of identifying thousands of images from their training set that include small animals as obstacles, yet these labels were not in the ground truth of their training data. Specifically, the team needed to identify unlabeled images from their training set that include:
- Pedestrians in unusual postures (~60 found with Nuro’s internal tool vs. 1000+ with Nucleus)
- Animal cases—horses, large birds, small non-pet animals (~50 found with Nuro’s internal tool vs. 400+ with Nucleus)
- Occluded and backlit pedestrians (10-20 found with Nuro’s internal tool vs. 500+ with Nucleus)
- Infrequently encountered vehicles such as excavators (10-20 found with Nuro’s internal tool vs. 500+ with Nucleus)
Yet these labels were not present in the ground truth of their training data.
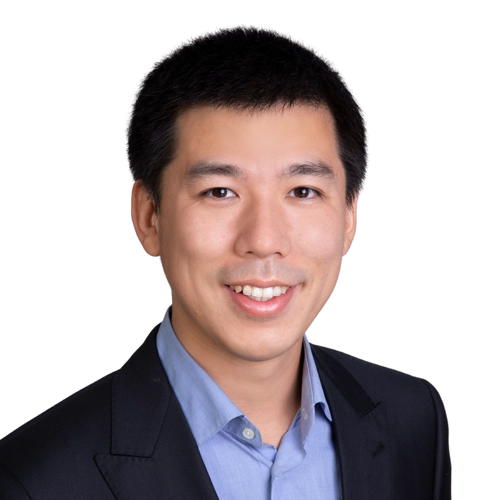
The Solution
Nucleus Helps Nuro’s Perception Team Collaborate to Unearth Safety-Critical Edge Cases.
Nuro aims to ingest a substantial portion of its training dataset into Nucleus to identify rare edge cases from a massive collected dataset with minimal effort or intervention from its ML team. Thus, Nuro can build robust, accurate models that can even handle rare but significant (or even dangerous) edge cases, like a sidewalk collision with a toddler or domestic animals.
Together, Nucleus’ Object Autotag and data curation workflows enable ML engineers to use a consistent platform for these common modeling tasks. Autotag lets users select unlabeled images of a certain category, and then using an internal model and its feature vectors, provide a set of similar images, suitable to be sent out for labeling with new class labels. That way, team members can all collaborate on the same unified platform. Nuro’s ML team can now incorporate newly identified edge cases (rare scenarios) and re-train a new model in just a few days rather than over a week.
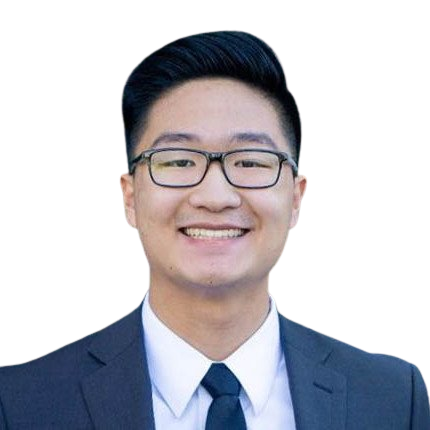
The Result
With Nucleus, Nuro Tackles the Ever Growing Long Tail of Rare Scenarios.
Today, Nuro’s Perception Team maintains an impressive dataset at a scale of over 500 million images. For training data, when the team holds the amount of labeled data fixed for supervised learning, they’ve been able to handle more and more edge cases like avoiding collisions with large birds. Thus, Nucleus helps Nuro achieve targeted levels of performance on specific subdomains.
Looking forward, Nuro intends to continue to scale up the size of its dataset that it curates with Nucleus so that the perception team will be ready to target any new edge cases, should they appear in the suburban wild. Future training data will likely include changing environmental conditions such as snow; noisy obstacles like tree, lawn, and leaf clippings; and identifying actions, such as the hand-wave of a pedestrian.
After re-training their models with data and labels that address these new scenarios, Nuro can improve the safety and efficiency of their automated deliveries with fewer human interventions required.
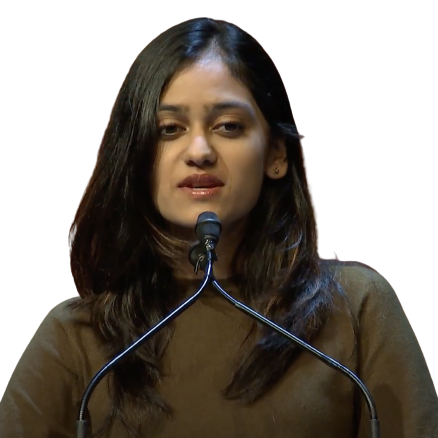