
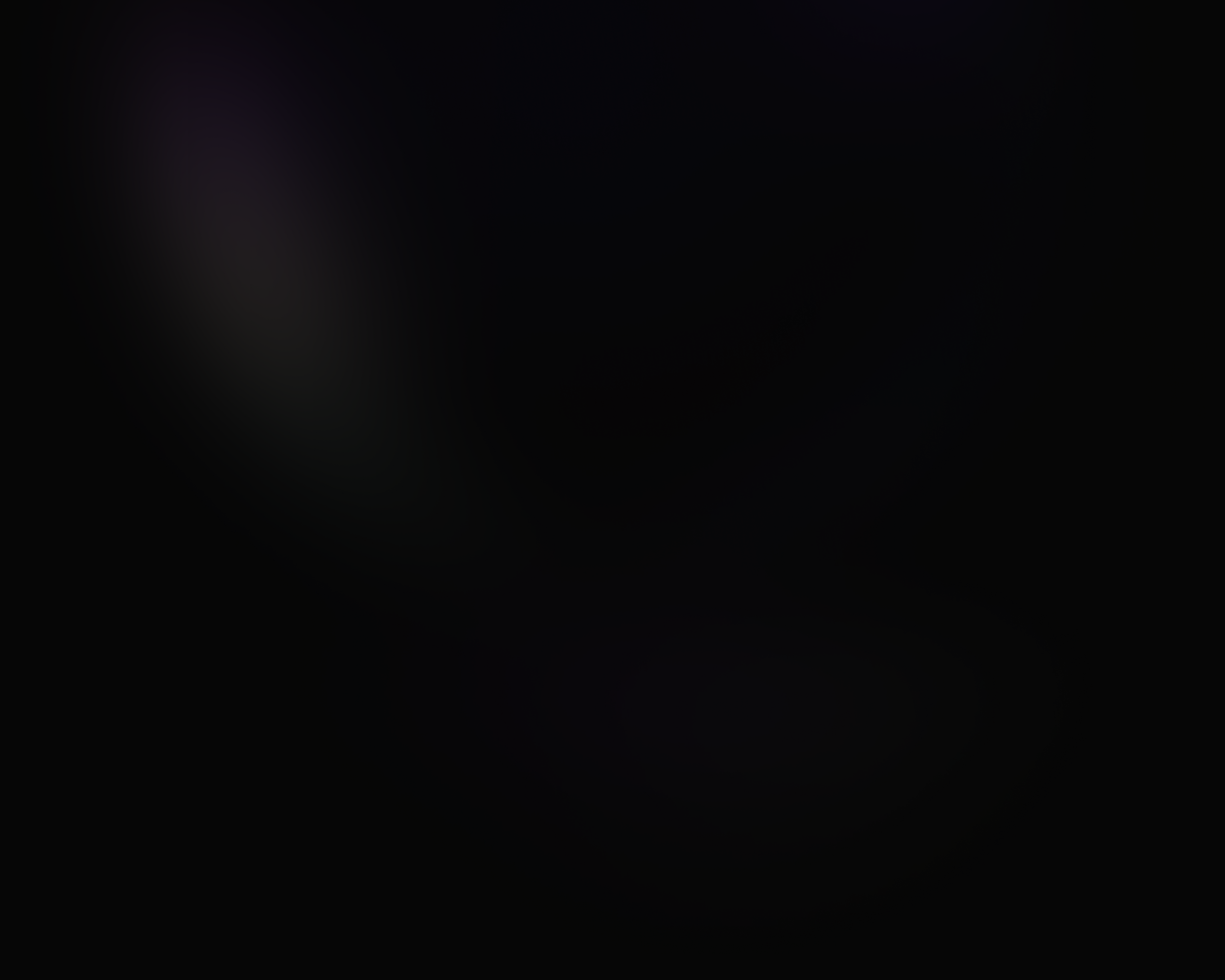
Overview
Nucleus Helps Velodyne Select Relevant Training Data Faster
Velodyne builds lidar sensors to enable safe navigation and autonomy. Velodyne’s revolutionary sensor and software solutions provide flexibility, quality, and performance to meet the needs of a wide range of industries, including robotics, industrial, infrastructure, and automotive.
Velodyne’s lidars generate a high-quality point cloud in a wide variety of light and weather conditions, with advanced sensor-to-sensor interference mitigation, power efficiency, and thermal performance. Velodyne uses Scale Nucleus to sort through edge cases in 3D sensor fusion data, which enables their machine learning (ML) team to train models on even rare scenarios such as:
- a warehouse employee storing boxes on top of a scissor lift.
- a warehouse employee carrying a large pallet.
- a person bending down as if to tie their shoes.
Nucleus reduced training data selection times for the data team by 50%.
The Problem
Finding the Right Toolset for Data Selection and Dataset Management
Velodyne Lidar’s data team collects large quantities of sensor data but finding a subset useful for training is challenging. The team was looking for an out-of-the-box vendor to provide the tools for data selection and management.
Common indoor robotics scenes were fairly easy to classify due to these scenarios comprising large portions of the datasets captured on their test robots. However, rarer scenarios, such as a warehouse employee stacking boxes on the top of a scissor lift, were relatively difficult to find.
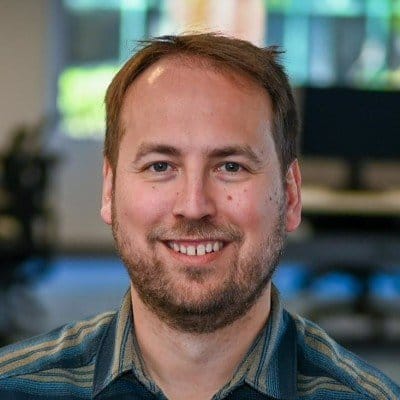
The Solution
Using Object Autotag to Cluster 3D Scenes by Querying 2D Images
The ML team captures image data along with the lidar point clouds and uses the Object Autotag feature in Scale Nucleus to query, browse and find similar images. These images are tied to 3D point clouds in a scene, enabling Velodyne to capture only point clouds of interest.
Through point cloud scenes, Nucleus supports multimodal data. It’s possible to search 2D image data using the model zoo built into Nucleus, Object Autotag and even natural language text queries to retrieve edge cases or exceptional examples in the associated 3D lidar data.
The Result
Efficient Training on New 3D Datasets to Detect New Classes
Velodyne's data team is now able to efficiently curate new datasets. While curating a 3D lidar dataset previously took days or even weeks, the Velodyne team was able to drill down on edge cases in a matter of hours, reducing the dataset creation step and kicking off their annotation job days earlier. As their library grows, they can easily refine ground truth and quickly identify new edge cases that their models are struggling with.
More broadly, companies worldwide are automating their supply chains to save money, improve efficiencies and alleviate bottlenecks. In warehouses, Velodyne's customers are using their lidar-equipped robotics for accurate object detection, classification, and path estimation.
This enables companies to move goods with a high level of precision, efficiency, and safety, helping to maintain continuity of operations. Currently, e-commerce retailers and sidewalk robotics programs are deploying Velodyne's lidar for fulfillment, delivery, and data-center operations.
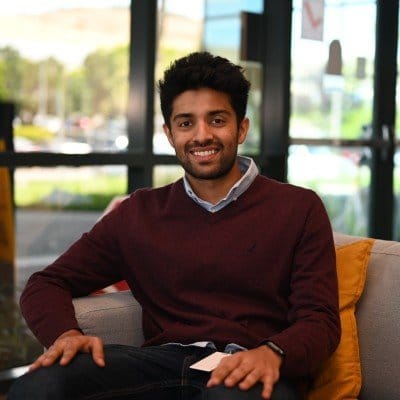