PandaSet
Public large-scale dataset for autonomous driving provided by Hesai & Scale. It enables researchers to study challenging urban driving situations using the full sensor suit of a real self-driving-car.
Overview
Sophisticated LiDAR technology meets high-quality data annotation
PandaSet aims to promote and advance research and development in autonomous driving and machine learning. The first open-source dataset made available for both academic and commercial use, PandaSet combines Hesai’s best-in-class LiDAR sensors with Scale AI’s high-quality data annotation. PandaSet features data collected using a forward-facing LiDAR with image-like resolution (PandarGT) as well as a mechanical spinning LiDAR (Pandar64). The collected data was annotated with a combination of cuboid and segmentation annotation (Scale 3D Sensor Fusion Segmentation). It features:
Data Collection
Complex Driving Scenarios in Urban Environments
For PandaSet we carefully planned routes and selected scenes that would showcase complex urban driving scenarios, including steep hills, construction, dense traffic and pedestrians, and a variety of times of day and lighting conditions in the morning, afternoon, dusk and evening. PandaSet scenes are selected from 2 routes in Silicon Valley: (1) San Francisco; and (2) El Camino Real from Palo Alto to San Mateo.
Car Setup
Vehicle, Sensor and Camera Details
We collected data using a Chrysler Pacifica minivan mounted with a suite of cameras and Hesai LiDARs.
- 5
- 10 Hz capture frequency
- 1/2.7” CMOS sensor of 1920x1080 resolution
- Images are unpacked to YUV 4:4:4 format and compressed to JPEG
Wide-Angle Cameras - 1
- 10 Hz capture frequency
- 1/2.7” CMOS sensor of 1920x1080 resolution
- Images are unpacked to YUV 4:4:4 format and compressed to JPEG
Long-Focus Camera - 1
- 1x Spinning LiDARs
- 64 channels
- 200m range @ 10% reflectivity
- 360° horizontal FOV; 40° vertical FOV (-25° to +15°)
- 0.2° horizontal angular resolution (10 Hz); 0.167° vertical angular resolution (finest)
- 10 Hz capture frequency
Pandar64: Mechanical Spinning LiDAR - 1
- Equivalent to 150 channels at 10 Hz
- 300m range @ 10% reflectivity
- 60° horizontal FOV; 20° vertical FOV (-10° to +10° with ±5° offset, configurable)
- 0.1° horizontal angular resolution; 0.07° vertical angular resolution (finest) at 10 Hz
- 10 Hz capture frequency
PandarGT: Solid-State LiDAR
PandarGT Road Test
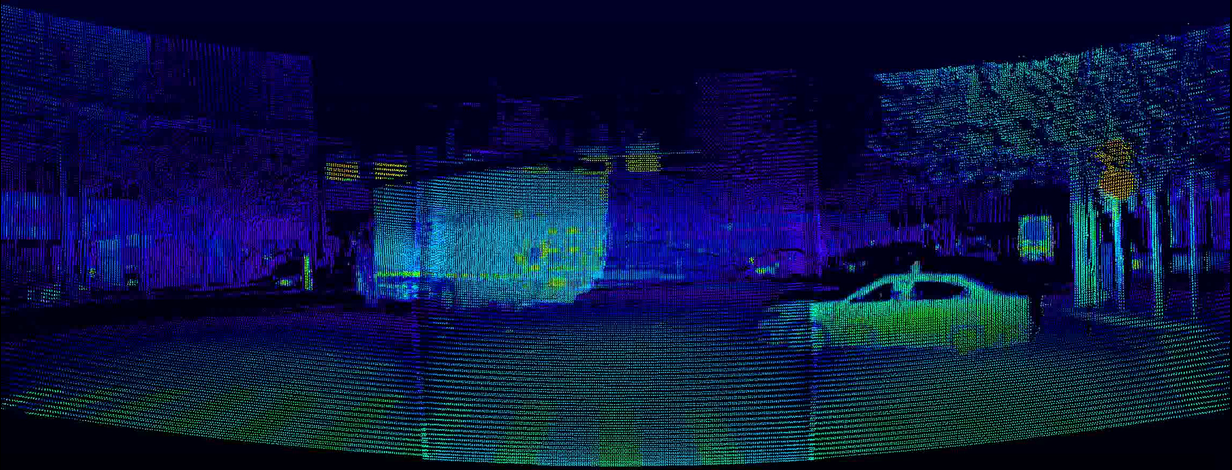
Sensor Calibration
Data alignment between sensors and cameras
To achieve a high quality multi-sensor dataset, it is essential to calibrate the extrinsics and intrinsics of every sensor. We express extrinsic coordinates relative to the ego frame, i.e. the midpoint of the rear vehicle axle. The most relevant steps are described below:
LiDAR extrinsics
Camera extrinsics
Camera intrinsic calibration
IMU extrinsics
Data Annotation
Complex Label Taxonomy
Scale’s data annotation platform combines human work and review with smart tools, statistical confidence checks and machine learning checks to ensure the quality of annotations. The resulting accuracy is consistently higher than what a human or synthetic labeling approach can achieve independently as measured against seven rigorous quality areas for each annotation. PandaSet includes 3D Bounding boxes for 28 object classes and a rich set of class attributes related to activity, visibility, location, pose. The dataset also includes Point Cloud Segmentation with 37 semantic labels including for smoke, car exhaust, vegetation, and driveable surface.